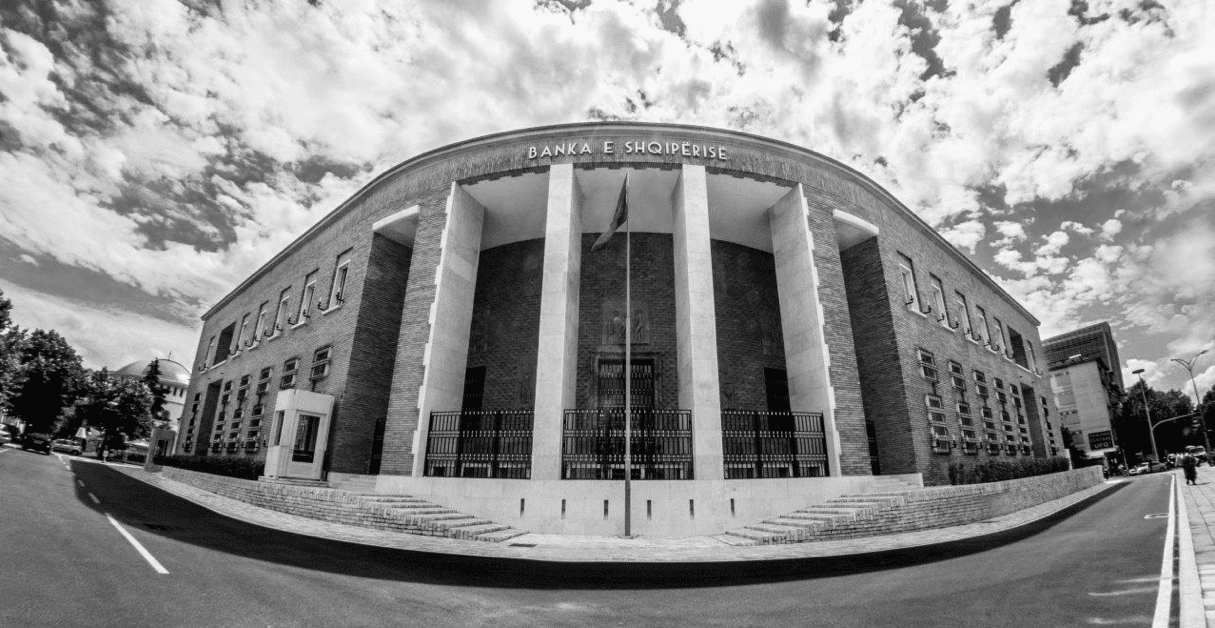
How to improve inflation forecasts: a Bayesian VAR approach for Albania
Forecasting the Albanian inflation through a Bayesian VAR model
Meri Papavangjeli, Bank of Albania
Generating accurate and reliable forecasts for inflation is a necessity for meeting a central bank’s primary objective of achieving and maintaining price stability. This issue takes even a greater importance considering that monetary policy effects on the economy are transmitted with time lags depending on the responsiveness of financial markets and real economy to policy interventions. Forecasting inflation is not only an important decision-making tool, but also serves as a crucial communication device. Monetary policy has become considerably more transparent over the recent decades. Open communication is beneficial to the stability and predictability of its transmission into the economy, and essential for the accountability of an independent central bank.
In a study conducted through the BCC programme, I analyse the appropriateness of using a Bayesian VAR (BVAR) model to forecast inflation in Albania. Unlike standard autoregressive vector models (VAR), the BVAR allows for the flexible inclusion of a broad range of variables, leading to a more comprehensive explanation of inflation. The BVAR is designed as a medium-sized model, which describes the most important dynamics and interactions between the determinants of inflation in Albania, taking into account developments in the real private sector, the financial sector and the foreign sector. It includes 9 variables (6 domestic and 3 foreign) at a quarterly frequency from 2002Q2 to 2018Q4. A central feature of Bayesian methods, including the BVAR, is to include prior information that the researcher has about the behaviour of the data, these priors are then combined with the data to construct the so-called posterior estimates. The two most common priors found in the literature are the Litterman-Minnesota and Normal-Wishart which are used for the estimation of the BVAR.[1]
The results from the BVAR are contrasted against the ones from several simpler econometric models. These include univariate models (the unconditional mean, a random walk, an autoregressive integrated moving average) and an unrestricted VAR. The best performing among these alternatives, namely the random walk and VAR, are used as benchmark against which we evaluate the forecast performance of the BVAR model. The forecasting performance of the various models is measured by the root mean squared error (RMSE) of the out-of-sample forecasts for forecast horizons up to 6 quarters. The forecasts are obtained in two ways (rolling and recursive forecasts) and compared to each-other for different time horizons. In addition, the accuracy of density forecast for both the BVAR and the benchmarks is measured by the average logarithmic scores and they are compared to each other.
The estimated results show that the BVAR approach, which incorporates more economic information, outperforms the benchmark univariate models and the VAR in different time horizons of the forecast sample using both forecast strategies. However, the improvement is moderate as the differences between the various models’ forecast performance are not statistically significant. Of course, this assessment could be revised once we can rely on longer samples of data.
A room for future work is to follow a forecast averaging approach, which would be stronger than the BVAR model studied in the paper. In my study, I do not compute forecast averages. This averaging procedure takes different variants of the BVAR that only differ in the prior views on the dynamics of inflation that the researcher inputs in the model (the variants however have the same likelihood function). Such an approach will allow us to consider different a priori views on the inflation process, and contrast their impact on the forecasting of inflation in a well-structured way. As different priors can all be reasonable, averaging over the various variants of the BVAR is likely to give stronger results than selecting only one of them. A forecast combination procedure of the BVAR with other short-term inflation forecasting models could be a successful strategy to improve forecast performance. By combining many misspecified models each incorporating information from different variables, model averaging usually outperforms forecasts from individual models (Aiolfi et al., 2011).
[1] An optimization procedure is implemented to select the best possible combination of the hyperparameters that characterize the priors in such a way that they maximize the marginal likelihood of the model.