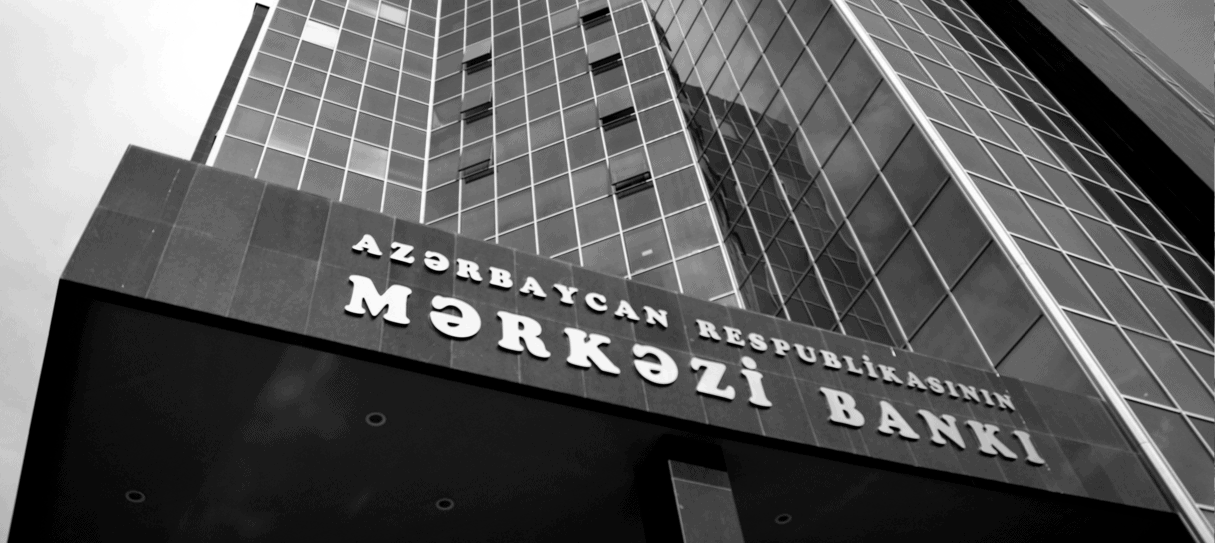
Do Factor Augmented VAR models always improve forecasts? The case of Azerbaijan
Vugar Rahimov, Central Bank of Azerbaidjan
Forecasting GDP and inflation is an important part of central bank decision-making, providing inputs for proactive policy formulation. Policy institutions use different forecasting models, going from simple univariate models to more complicated multivariate ones. The proper use of models greatly depends on data availability and data quality. This is an important dimension for many emerging and developing countries that often lack access to high quality data. In contrast, researchers and forecasters in advanced countries benefit from high frequency long time series data in broad area, from micro to macro level.
A standard forecasting framework combining several variables is the vector autoregression (VAR) approach developed by Christopher Sims in the 1980’s. This model consists of a system of equations in which each variable is impacted by its own lags as well as the current values and the lags of other variables. A shortcoming of the approach is that it cannot consider more than a relatively small number of variables. Factor models represent another type of econometric approach and have gained popularity in early 2000s, after works done by Stock and Watson (2002a, 2002b). The central point is to combine a broad range of indicators of the same economic concept (such as different measures of inflation) and extract a factor that is common to them, instead of focusing on one specific variable. Bernanke et al. (2005) have combined the two approaches and developed a factor-augmented VAR (FAVAR) model.
Since then, this method has been widely used in macroeconomics, particularly, in data-rich environment. The FAVAR approach goes around the inability of standard VAR models to include a broad range of data, and helps handling omitted variable bias, as well as providing some robustness in the presence of structural breaks and requires minimal conditions on the errors.
In Rahimov et al. (2019), we build a FAVAR model to forecast inflation and output in Azerbaijan. We contrast the model forecasting ability against other simpler models. The main challenge in this study is the limited quality of data and a short sample (starting from the first quarter of 2003). The variables of interest also exhibit high seasonality and volatility, and include two major structural breaks (in 2007-2008 and in 2015-2016). The number of indicators in some areas, such as labor market and government finance, is also limited. We nonetheless manage to collect 77 quarterly series of indicators on the real sector, prices, monetary variables, government finances and the labor market. We split them into two groups based on their correlations with interest variables (i.e., inflation and GDP growth), and extract three principal components for each group that is included in our FAVAR model.
The forecast ability of the model is assessed using the root mean squared error (RMSE). We show that univariate models outperform the FAVAR in the forecasting of inflation, particularly at a short horizon. Diebold-Mariano (DM) test confirms that the differences in RMSEs between models are significant in short-term horizons. By contrast, FAVAR performs better than univariate models in the forecasting of GDP, even though the DM tests indicate that the differences in RMSE between the models are not significant. The results can partly be attributed to the challenging structure of the variables (short samples, the presence of structural breaks occurred during this period, limited data quality of the indicators used in constructing the factors). The strong inertial component in many
variables – particularly, inflation –could be another reason for superior performance of the univariate model for inflation forecasting.
In summary, our study reveals that FAVAR models do not always produce best forecasts, and researchers should use care in selecting specific models used in forecasting. While having a rich dataset covering a broad range of indicators helps getting better forecasts, other characteristics, such as data quality, length and frequency of data, are more important.